Revolutionizing Agriculture: Large-Scale Applications of Machine Learning and Remote Sensing
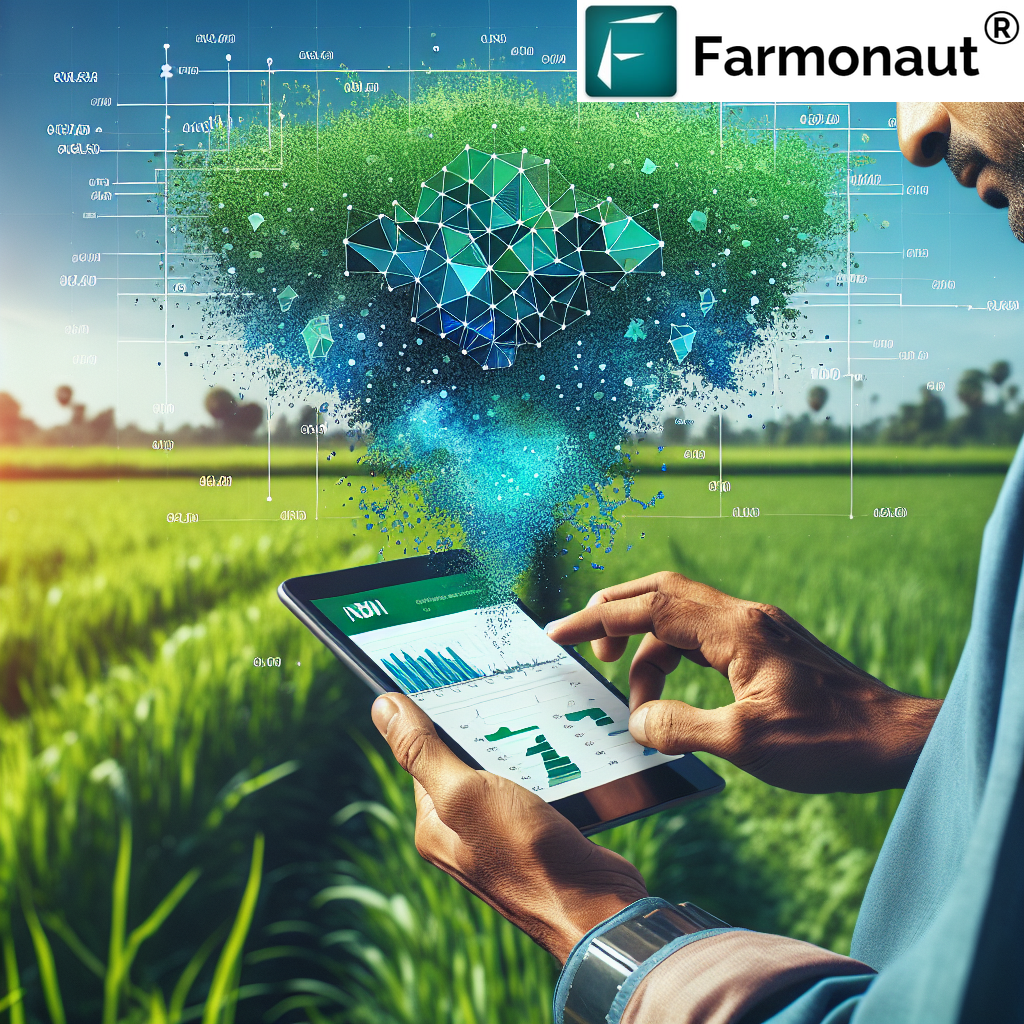
In the ever-evolving landscape of agriculture, we at Farmonaut are at the forefront of leveraging cutting-edge technologies to revolutionize farming practices. Our focus on large scale applications of machine learning using remote sensing for building agriculture solutions has positioned us as pioneers in the agritech industry. This comprehensive blog post delves into the intricate world of remote sensing, machine learning, and their transformative impact on agriculture.
Understanding Remote Sensing in Agriculture
Remote sensing has emerged as a game-changing technology in modern agriculture. It involves the collection of data about objects or areas from a distance, typically using satellites or aerial imagery. This non-invasive method of gathering information has proven invaluable in addressing various sensing problems in agriculture.
Key Benefits of Remote Sensing in Agriculture:
- Large-scale crop monitoring
- Early detection of plant stress and diseases
- Precision agriculture practices
- Resource optimization
- Environmental impact assessment
At Farmonaut, we harness the power of remote sensing to provide farmers with actionable insights, enabling them to make informed decisions about their crops and land management practices.
The Role of Machine Learning in Agricultural Remote Sensing
Machine learning algorithms play a crucial role in interpreting the vast amounts of data collected through remote sensing. These algorithms can identify patterns, predict outcomes, and provide valuable insights that would be impossible for humans to discern manually.
Applications of Machine Learning in Agricultural Remote Sensing:
- Crop classification and mapping
- Yield prediction
- Pest and disease detection
- Soil moisture estimation
- Land use change detection
Our Jeevn AI Advisory System at Farmonaut is a prime example of how we integrate machine learning with remote sensing data to provide personalized farm management recommendations.
NDWI: A Crucial Index in Remote Sensing for Agriculture
One of the most frequently asked questions in our field is, “What is NDWI in remote sensing?” NDWI, or Normalized Difference Water Index, is a remote sensing-derived index that helps in assessing water content in vegetation and soil moisture levels.
Understanding NDWI:
NDWI is calculated using near-infrared (NIR) and short-wave infrared (SWIR) bands of satellite imagery. The formula is:
NDWI = (NIR – SWIR) / (NIR + SWIR)
This index is particularly useful for:
- Monitoring drought conditions
- Assessing irrigation needs
- Detecting water stress in crops
- Mapping water bodies and wetlands
At Farmonaut, we incorporate NDWI analysis into our satellite-based crop health monitoring system, providing farmers with crucial information about their crops’ water status.
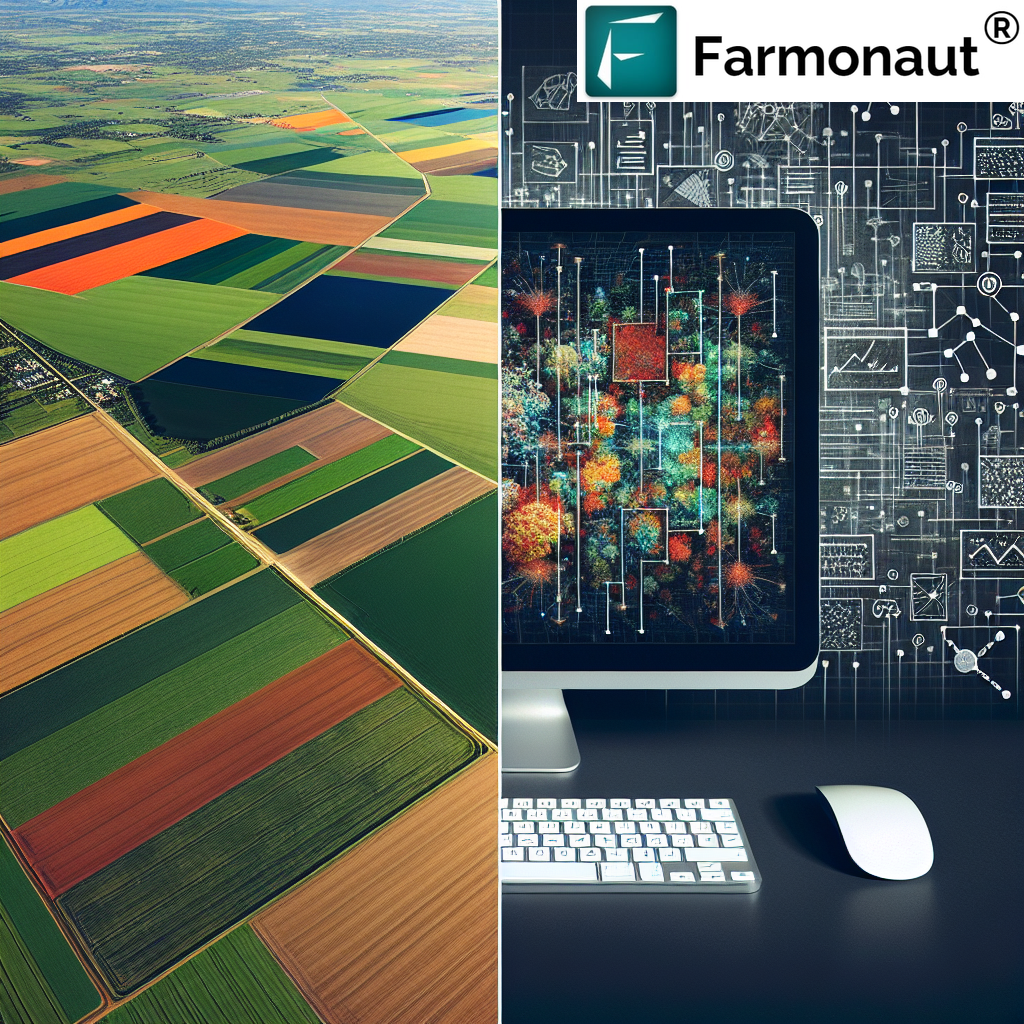
Large-Scale Applications of Machine Learning in Agriculture
The integration of machine learning and remote sensing has paved the way for large scale applications of machine learning using remote sensing for building agriculture solutions. These applications are transforming the agricultural landscape on a global scale.
Key Large-Scale Applications:
- Regional Crop Yield Forecasting: Machine learning models can analyze satellite imagery and historical data to predict crop yields across vast regions, aiding in food security planning and market predictions.
- Precision Agriculture at Scale: By analyzing high-resolution satellite imagery, machine learning algorithms can generate precise prescription maps for variable rate applications of fertilizers and pesticides across large farms.
- Automated Crop Type Mapping: Machine learning classifiers can accurately identify and map different crop types over large areas, providing valuable information for agricultural policy-making and land use planning.
- Climate Change Impact Assessment: By analyzing long-term satellite data, machine learning models can assess the impact of climate change on agricultural productivity and help in developing adaptation strategies.
- Large-Scale Soil Health Monitoring: Machine learning algorithms can process multispectral satellite imagery to assess soil health parameters across vast agricultural regions, aiding in sustainable land management practices.
At Farmonaut, we’re constantly working on refining and expanding these large-scale applications to provide more value to our users, from individual farmers to government agencies.
Addressing Sensing Problems in Agriculture
While remote sensing offers numerous benefits, it also comes with its own set of challenges or sensing problems. These issues need to be addressed to ensure the accuracy and reliability of the data and insights generated.
Common Sensing Problems and Solutions:
- Atmospheric Interference: Clouds, haze, and atmospheric particles can interfere with satellite imagery. We use advanced atmospheric correction algorithms and multi-temporal image compositing to mitigate these effects.
- Spatial Resolution Limitations: Some satellites may not provide high enough resolution for certain applications. We combine data from multiple satellite sources and use super-resolution techniques to enhance image quality.
- Temporal Resolution Gaps: Infrequent satellite passes can lead to gaps in data. Our system integrates data from multiple satellites and uses interpolation techniques to provide more continuous monitoring.
- Spectral Mixing: Mixed pixels containing multiple land cover types can complicate analysis. We employ spectral unmixing algorithms and sub-pixel analysis to address this issue.
- Ground Truth Data Scarcity: Limited ground-based data can affect model accuracy. We’ve developed a collaborative platform that allows farmers to contribute ground truth data, improving our models over time.
By continuously innovating and refining our approaches, we at Farmonaut strive to overcome these sensing problems and provide the most accurate and reliable agricultural insights possible.
The Farmonaut Advantage: Satellite vs. Drone and IoT Monitoring
While there are various technologies available for farm monitoring, our satellite-based system at Farmonaut offers distinct advantages over drone and IoT-based solutions. Here’s a comparison:
Feature | Farmonaut Satellite System | Drone-based Monitoring | IoT-based Monitoring |
---|---|---|---|
Coverage Area | Large (Global) | Medium | Small (Local) |
Frequency of Data Collection | Regular (Every few days) | On-demand | Continuous |
Initial Setup Cost | Low | High | Medium to High |
Operational Complexity | Low | High | Medium |
Data Processing Time | Fast | Medium | Real-time |
Scalability | Highly Scalable | Moderately Scalable | Limited Scalability |
Weather Dependency | Moderate | High | Low |
Multi-spectral Analysis | Yes | Yes (with specialized equipment) | Limited |
As evident from the comparison, our satellite-based system offers a unique combination of large-scale coverage, cost-effectiveness, and ease of use that makes it ideal for a wide range of agricultural applications.
The Future of Remote Sensing and Machine Learning in Agriculture
As we look to the future, the potential for large scale applications of machine learning using remote sensing for building agriculture solutions continues to expand. Some exciting developments on the horizon include:
- Hyperspectral Imaging: Future satellites with hyperspectral capabilities will provide even more detailed information about crop health and soil conditions.
- Integration with IoT: Combining satellite data with ground-based IoT sensors will create more comprehensive and accurate monitoring systems.
- Advanced AI Models: Developments in deep learning and computer vision will lead to more sophisticated crop analysis and prediction models.
- Real-time Monitoring: Improvements in satellite technology and data processing will enable near real-time monitoring of agricultural areas.
- Precision Agriculture 2.0: The next generation of precision agriculture will leverage AI to automate many farm management decisions.
At Farmonaut, we’re committed to staying at the forefront of these technological advancements, continually improving our services to provide the best possible solutions for our users.
Conclusion: Empowering Agriculture Through Technology
The integration of remote sensing and machine learning is ushering in a new era of data-driven, precision agriculture. From addressing sensing problems to leveraging indices like NDWI in remote sensing, these technologies are providing farmers with unprecedented insights into their crops and land.
At Farmonaut, we’re proud to be at the forefront of this agricultural revolution. Our commitment to developing large scale applications of machine learning using remote sensing for building agriculture solutions is helping farmers around the world increase productivity, reduce resource use, and adopt more sustainable practices.
We invite you to join us on this journey towards a more efficient and sustainable agricultural future. Explore our services and see how Farmonaut can transform your farming practices:
- Try our app: Farmonaut Mobile App
- Integrate our API: Farmonaut API
- Download on Google Play: Farmonaut for Android
- Download on App Store: Farmonaut for iOS
- Developer Documentation: Farmonaut API Docs
Subscribe to Farmonaut
Frequently Asked Questions (FAQ)
-
Q: What is remote sensing in agriculture?
A: Remote sensing in agriculture refers to the practice of gathering information about crops and farmland from a distance, typically using satellite or aerial imagery. It allows farmers and agricultural experts to monitor large areas efficiently, assess crop health, predict yields, and make informed decisions about farm management. -
Q: How does machine learning enhance remote sensing in agriculture?
A: Machine learning algorithms can analyze vast amounts of remote sensing data quickly and accurately. They can identify patterns, classify crop types, detect anomalies, predict yields, and provide insights that would be difficult or impossible for humans to discern manually. This enhances the value and applicability of remote sensing data in agricultural decision-making. -
Q: What is NDWI and why is it important in agriculture?
A: NDWI stands for Normalized Difference Water Index. It’s a remote sensing-derived index that helps assess water content in vegetation and soil moisture levels. NDWI is important in agriculture because it helps monitor drought conditions, assess irrigation needs, detect water stress in crops, and map water bodies. This information is crucial for effective water management in farming. -
Q: How does Farmonaut’s satellite-based system compare to drone or IoT-based monitoring?
A: Farmonaut’s satellite-based system offers several advantages over drone or IoT-based monitoring. It provides large-scale coverage (global), requires low initial setup costs, has low operational complexity, and is highly scalable. While it may not offer the real-time data of IoT systems or the on-demand flexibility of drones, it provides a cost-effective solution for regular, large-scale farm monitoring. -
Q: What are some common sensing problems in agricultural remote sensing?
A: Common sensing problems include atmospheric interference (clouds, haze), spatial resolution limitations, temporal resolution gaps, spectral mixing, and scarcity of ground truth data. These issues can affect the accuracy and reliability of remote sensing data and require sophisticated techniques to address. -
Q: How is Farmonaut addressing these sensing problems?
A: Farmonaut addresses these problems through various means, including using advanced atmospheric correction algorithms, combining data from multiple satellite sources, employing interpolation techniques, using spectral unmixing algorithms, and developing a collaborative platform for ground truth data collection. -
Q: What are some large-scale applications of machine learning in agricultural remote sensing?
A: Large-scale applications include regional crop yield forecasting, precision agriculture at scale, automated crop type mapping, climate change impact assessment, and large-scale soil health monitoring. These applications leverage machine learning algorithms to analyze vast amounts of satellite data and provide insights across large agricultural regions. -
Q: How can farmers benefit from Farmonaut’s services?
A: Farmers can benefit from Farmonaut’s services in several ways:- Real-time crop health monitoring
- Personalized farm management recommendations
- Early detection of crop stress and diseases
- Optimized resource use (water, fertilizers, pesticides)
- Yield prediction and harvest planning
- Access to historical farm data for long-term planning
-
Q: Is Farmonaut’s technology suitable for small-scale farmers?
A: Yes, Farmonaut’s technology is designed to be accessible and beneficial for farmers of all scales, including small-scale farmers. Our satellite-based system requires no on-farm equipment installation, making it a cost-effective solution for smaller operations. -
Q: What’s the future of remote sensing and machine learning in agriculture?
A: The future of these technologies in agriculture is promising, with developments expected in hyperspectral imaging, integration with IoT devices, more advanced AI models, near real-time monitoring capabilities, and the next generation of precision agriculture that leverages AI for automated decision-making.
We hope this comprehensive guide has provided valuable insights into the world of remote sensing and machine learning in agriculture. At Farmonaut, we’re committed to harnessing these technologies to empower farmers and revolutionize agricultural practices. Join us in shaping the future of farming!